Kristina Lerman is a Principal Scientist at the Information Sciences Institute and holds a joint appointment as a Research Professor in the USC Viterbi School of Engineering's Computer Science Department. Her research focuses on applying network- and machine learning-based methods to problems in social computing.
Lerman’s research aims to harness the power of AI in a connected world. Her past work has helped shape how we think about social media platforms, elucidating the role of social networks in the spread of information and also what we see and what we know. Her current research examines digital vulnerabilities in a hyperconnected world, including misinformation, online influence campaigns and polarization. Lerman’s second major research area focuses on how AI systems can learn from biased data. Her group is developing statistical methods to audit biases in real-world data and create fair models from biased data. These models will, in turn, enable socially-embedded AI systems to mediate human interactions to reduce antisocial behaviors and mitigate their unintended harms.
Selected Publications
Lerman, K., Yu, Y., Morstatter, F., & Pujara, J. (2022). Gendered citation patterns among the scientific elite. Proceedings of the National Academy of Sciences, 119 (40), e2206070119.
Mehrabi, N., Morstatter, F., Saxena, N., Lerman, K., & Galstyan, A. (2021). A survey on bias and fairness in machine learning . ACM Computing Surveys (CSUR) 54 (6), 1-35
Lerman, K. (2018). Computational social scientist beware: Simpson’s paradox in behavioral data. Journal of Computational Social Science, 1(1), 49-58.
Lerman, K. (2016). Information is not a virus, and other consequences of human cognitive limits. Future Internet, 8(2), 21.
Lerman, K., Yan, X., & Wu, X. Z. (2016). The" majority illusion" in social networks. PloS one, 11(2), e0147617.
Research Interests
Socially-Embedded AI
Fairness in AI, bias in data, bias in networks, algorithmic bias.Social Networks
Complex systems, dynamic networks, mathematical analysis, multi-agent systems.Social Computing
Social web and social media, learning from social annotation, social dynamics.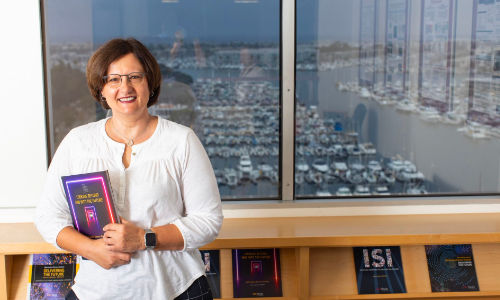