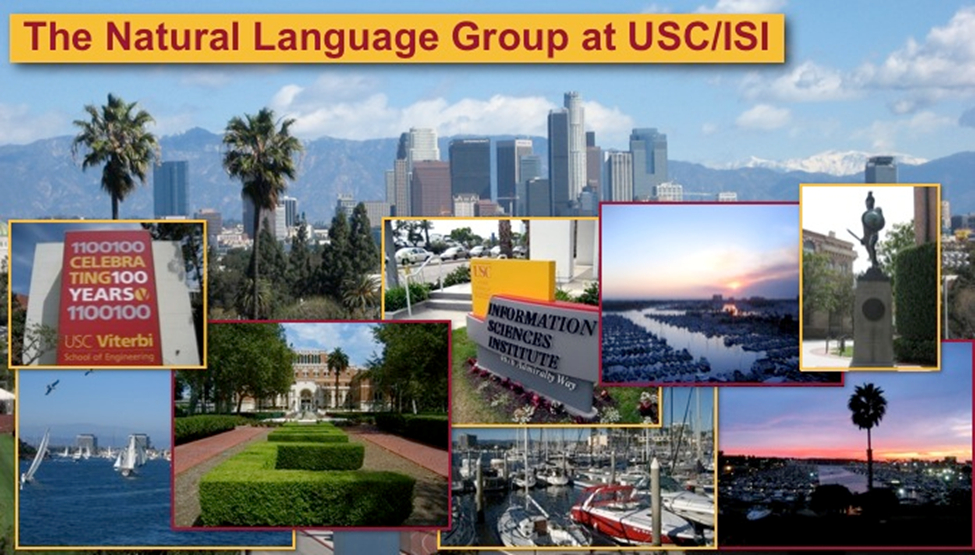
The Natural Language Group at the USC Information Sciences Institute conducts research in natural language processing and computational linguistics, developing new linguistic and mathematical techniques to make better technology. We have a wide range of ongoing projects, including those related to statistical machine translation, question answering, summarization, ontologies, information retrieval, and natural language generation. A high-quality group of faculty, research staff, and graduate students makes all these fascinating topics come to life.
The group is part of the larger computational linguistics community at USC.
The USC Information Sciences Institute is an academic research institute, part of the Andrew and Erna Viterbi School of Engineering. Many ISI researchers are also on the faculty in the Computer Science department, and likewise, many CS graduate students do their dissertation research here. ISI's Artificial Intelligence Division is one of the largest university-based artificial intelligence groups in the world.