Completed Projects
Completed Projects
- ALLAN: Agents Learning Lying And Negotiation
- AMR: Abstract Meaning Representation
- CLEAR: Cross-Lingual Event and Argument Retrieval
- CORAL: Combined Representations for Adept Learning
- CWIC: Communicating Intelligently with Computers
- DARMA: Dialogue Agent for Reducing Malicious Acts
- DECODE: Deciphering Historical Manuscripts
- Discerning Group Biases in Online Communities via Linguistic Analysis
- DIG: Domain-Specific Insight Graphs
- ELICIT: A System for Extracting and Organizing Causal Information
- ELISA: Exploiting Language Information for Situational Awareness
- EvidxExtraction: Evidence Extraction Systems for the Molecular Interaction Literature
- L2K2R2: Learning to Know to Read
- LESTAT: Learning Event Schema Temporally and Transmodally
- MICS: Machine Intelligence from Common Sense
- SARAL: Summarization and Domain-Adaptive Retrieval Across Languages
- Viola: Neural Generation with Improvised Dialogues and Common Sense Reasoning
ALLAN
Agents Learning Lying And Negotiation
Nearly every business transaction and every diplomatic agreement from haggling over a turnip at a farmers market to a multi-trillion dollar trade deal is a negotiation. These diplomatic arts are not just a series of cold calculations to optimize an objective function. They are built on cultural norms and emotional ties learned from human teachers and a history of past negotiations. If we want computers to help human negotiators and to hold their own against humans, the game of Diplomacy is an ideal testbed for teaching computer agents how to learn to negotiate. We will build agents that negotiate and cooperate in Diplomacy, a game of politics and war that relies on negotiated agreements between players that must ultimately be fluid, with lies and betrayal used to manipulate other players into temporarily behaving in helpful ways. To learn to play this game, which is notable for being beloved among political operatives like John F. Kennedy and Henry Kissinger, we will incorporate deep reinforcement learning, sophisticated strategy and tactics engines, and a dialogue model fine tuned to opportunistically lie. This work is a collaboration between the University of Maryland (Prime), Princeton University, and the University of Sydney.
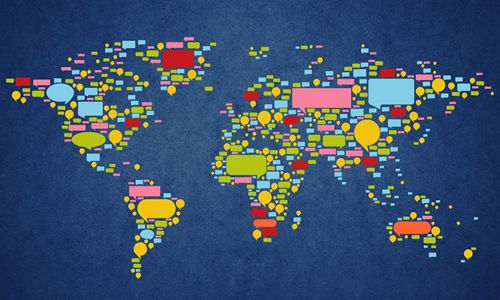
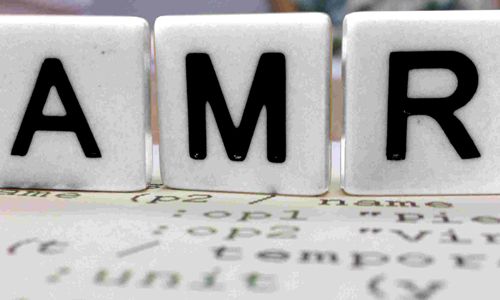
AMR
Abstract Meaning Representation
The AMR Bank is a set of English sentences paired with simple, readable semantic representations. We hope that it will spur new research in natural language understanding, generation, and translation. Please visit the AMR page for more details. Thanks to NSF (IIS-0908532) for funding the initial design of AMR, and to DARPA MRP (FA-8750-09-C-0179) for supporting a group to construct consensus annotations and the AMR Editor. The initial AMR bank was built under DARPA DEFT FA-8750-13-2-0045 (PI: Stephanie Strassel; co-PIs: Kevin Knight, Daniel Marcu, and Martha Palmer) and DARPA BOLT HR0011-12-C-0014 (PI: Kevin Knight).
CLEAR
Cross-Lingual Event and Argument Retrieval
The automatic extraction of events from text has empowered tasks as varied as the prediction of political stability forecasting or the automatic creation of in-depth biomedical information resources. However, most training data for event extraction models is available only in English. Under IARPA’s BETTER program, ISI researchers are developing an innovative end-to-end, cross-lingual system which will provide personalized, multi-lingual semantic extraction and retrieval from text in foreign languages, using only English training data. Our collaborator on this effort is UMass Amherst. This research is based upon work supported in part by the Office of the Director of National Intelligence (ODNI), Intelligence Advanced Research Projects Activity (IARPA), via 2019-19051600007.
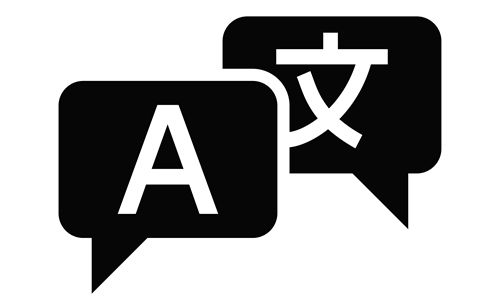
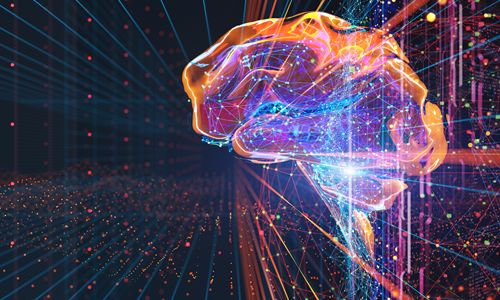
CORAL
Combined Representations for Adept Learning
Modern applications of machine learning (ML) constitute transformative technologies, whose adoption is spreading rapidly and impacting myriad sectors of the economy. However, ML’s revolutionary potential is limited by its cost—both the cost of creating corpora of labeled training data and the difficulty of adapting successful models to perform well in related domains. The goals of CORAL are to create revolutionary machine learning (ML) technology that will achieve state-of-the-art (SOTA) performance on a broad range of tasks, but require a factor of 106 less training data than the SOTA to automatically create a new capability for a new domain and task, and require 106 less training data to automatically adapt to a related domain. We additionally will develop and test information-theoretic techniques that characterize the limits of ML and adaptation. Our collaborators are the California Institute of Technology, Carnegie Mellon University, Columbia University, the NVIDIA Corporation, and the University of Illinois, Urbana-Champaign. This work is based in part on research sponsored by Air Force Research Laboratory (AFRL) under agreement number FA8750-19-1-1000.
CWIC
Communicating Intelligently with Computers
In this project, we work on human/robot communication, and on creative language generation. This work is supported by Contract W911NF-15-1-0543 with the US Defense Advanced Research Projects Agency (DARPA).
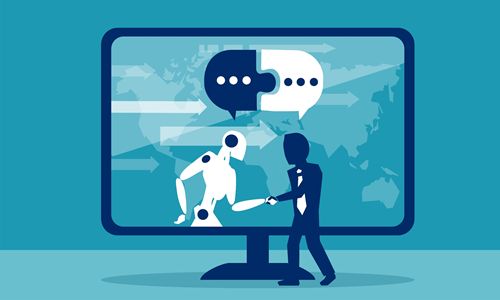
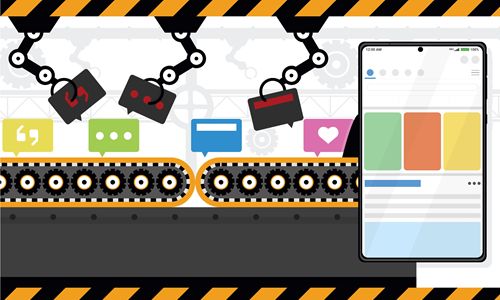
DARMA
Dialogue Agent for Reducing Malicious Acts
Social media has transformed how people share information and exchange ideas; however, unfettered communication has also unleashed toxic, anti-social behaviors, such as harrassment, disagreement, personal attacks, malicious rhetoric and other toxic communication acts. Without mediation, these anti-social behaviors create discord within a community and impede its ability to collaborate, share information, create consensus and build trust. We will build a multilingual, adaptable bots that mediate online dialogue so as to limit communication breakdown due to toxic behaviors and facilitate civil discourse. DARMA bots will monitor online conversations looking for indicators of toxic behaviors such as hate speech, trolling, and polarization. Our bots will determine the appropriate time to intervene, and will do so using principles of world-building and shared mental state learned from improvisational theater corpora and conflict resolution dialogues. DARMA will be able to operate in up to 500 different world languages (which cover more than 90% of primary languages of current world population) via our massively multilingual machine translation systems, which can be adapted to new domains using only monolingual adaptation data. This work is carried out with funding from DARPA (HR0011-22-9-0025).
DECODE
Deciphering Historical Manuscripts
In collaboration with colleagues at Uppsala University (Sweden), we are collecting enciphered manuscripts from the European modern era (1600-1800) and developing software to automatically decipher them. This work is supported by the Swedish Research Council and a gift from Google, Inc.
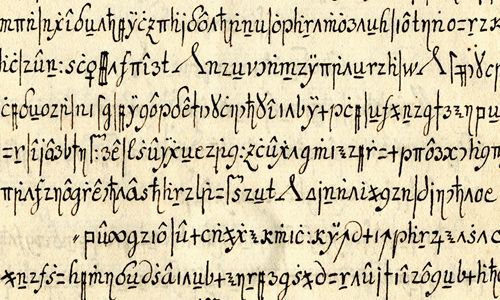
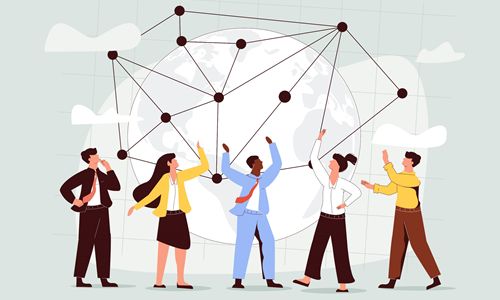
Discerning Group Biases in Online Communities via Linguistic Analysis
Cultural models are shared understandings of the world by particular cultures, which help individuals interpret the world around them. These models help provide people with internal mental heuristics for what is right, what is just, what is edible, among others. Understanding these models helps us to better understand the nature of the cultures which adopt them, as they provide rules for everyday life. The underlying mechanisms for these models are currently collected through ethnographic analysis undertaken by social scientists using specialized tools like interviews with key informants, pile sorts, and other techniques to better extract the underlying workings of a culture. While social scientists can use specialized tools like interviews with key informants, pile sorts, this “thick data” about the cultures being studied. It is cumbersome and time-consuming, and often requires months to years of fieldwork from social scientists to understand these models and can not easily scale across different types of stereotypes. Fortunately, these shared understandings are redundancies that can be exploited by machine learning techniques to quickly understand the underlying principles of a culture. In the past decade, enormous quantities of textual data, including product reviews, discussion forums, and digital contents, have been generated by online communities. This provides an opportunity and challenge for computer scientists and social scientists to study the underlying group biases present in the online content at scale. The primary scientific goal of the project is to develop a computational framework for rapid data-driven ethnographic analysis of online communities using statistical machine learning and computational linguistic tools. This work is carried out with funding from the DARPA UGB program, HR0011890019.
DIG
Domain-Specific Insight Graphs
DIG is a domain-specific indexing, search and analysis system. The DIG system harnesses state-of-the-art open source software combined with an open architecture and flexible set of APIs to facilitate the integration of a variety of extraction and analysis tools.
Please visit the DIG page for more details. This research is supported in part by the Defense Advanced Research Projects Agency (DARPA) and the Air Force Research Laboratory (AFRL) under contract number FA8750-14-C-0240, and in part by the National Science Foundation under Grant No. 1117913.
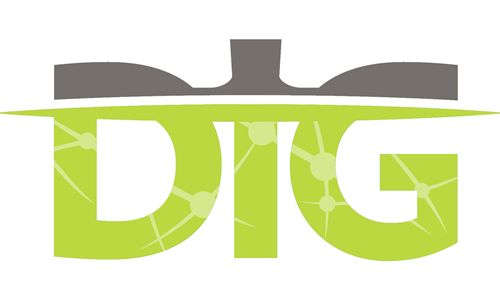
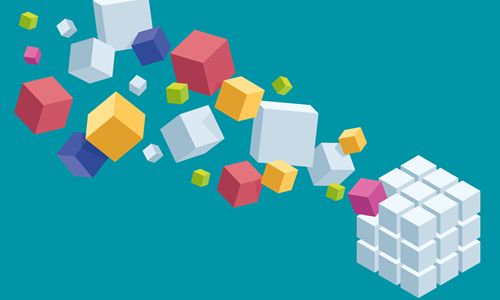
ELICIT
A System for Extracting and Organizing Causal Information
Optimal decision making requires ready access to disparate sources of structured (e.g., databases) and unstructured (e.g., natural language) information. ELICIT researchers are developing a framework that integrates concepts of causality, factual knowledge, and meta-reasoning into a model-driven knowledge graph representation that allows decision makers to access relevant knowledge. Teammates include Rensselaer Polytechnic Institute, CMU, and Lockheed Martin ATL. Sponsored by the Defense Advanced Research Projects Agency (DARPA) Causal Exploration program (FA8650-17-C-7715).
ELISA
Exploiting Language Information for Situational Awareness
Today's automatic parsers, translators, extractors, and dictionaries cover a tiny fraction of the world's languages. Can we use general knowledge of how language works to extend the reach of natural language tools? In this project, we develop technology for rapidly constructing information extraction (IE), machine translation (MT), and topic and sentiment processing capabilities for new languages. Our collaborators are ICSI, Brno University of Technology, University of Pennsylvania, University of Notre Dame, Rensselaer Polytechnic Institute, and Next Century, Inc. This work is carried out with funding from DARPA (HR0011-15-C-0115).
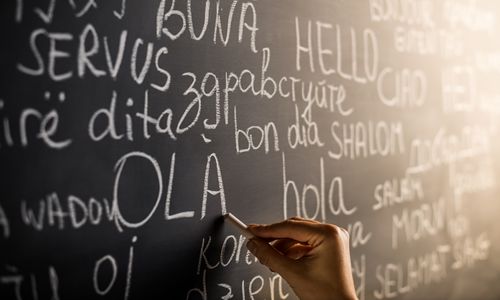
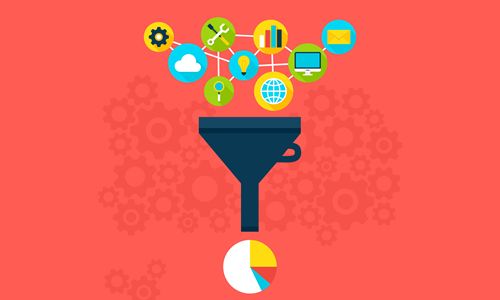
EvidxExtraction
Evidence Extraction Systems for the Molecular Interaction Literature
Biomedical databases describe the claims made by scientists in detail, but rarely provide descriptions of any supporting evidence that a consulting scientist could use to understand why the claims are being made. Currently, the process of curating evidence into databases is manual, time-consuming and expensive; thus, the evidence is recorded in papers but not generally captured in database systems. Although experimental evidence is complex, it conforms to certain principles of experimental design. Exploiting these principles has permitted us to devise a preliminary, robust, general-purpose representation for experimental evidence. A major goal of our project is that we will develop methods to extract this evidence from scientific papers automatically (1) by using natural language processing to read information from the text used by scientists to describe their results and (2) by using image processing on a specific subtype of figure that is common in molecular biology papers. We will develop these tools and package them so that they may then also be used for evidence pertaining to other areas of research in biomedicine. Funding statement: This work is carried out with funding from an NIH R01 (LM012592).
L2K2R2
Learning to Know to Read
Scientists are overwhelmed with scientific literature. If we can build machines to read scientific papers and understand them, we can help science move faster. This work is sponsored by DARPA Big Mechanism (W911NF-14-1-0364).
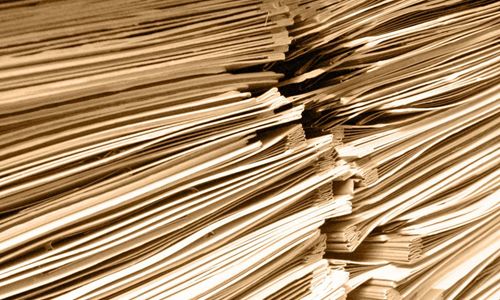
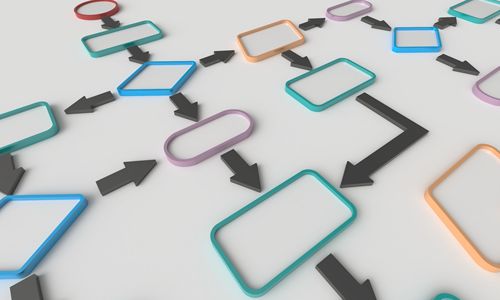
LESTAT
Learning Event Schema Temporally and Transmodally
Understanding a complex event requires organizing its typical sequence of actions and the pattern of duration and participation in these actions. By organizing events in this manner, downstream analytics (or analysts) are able to identify the early warning signs of a process and recognize important but missing information. While handcrafted models provide this capability for well-understood processes, a system that learns schemas from data offers the opportunity to extend event-focused analytics to new domains. We are developing the LESTAT system, which semi-automatically discovers schemas for complex events (CE). These automatically discovered schemas will be general, composable, and specializable to support domain-specific contexts. LESTAT’s schemas will be the basis for systems developed elsewhere to track and anticipate the activities of entities of interest based on detecting individually weak event signals in multi-modal, multilingual input. Our collaborators are Arizona State University and the University of Central Florida. This research is based upon work supported by DARPA's KAIROS program, Contract FA8750-19-2-0500.
MICS
Machine Intelligence from Common Sense
Our goal is to automatically learn a common sense repository (CSR) of knowledge that can be applied to diverse problems. In this project we learn common sense from textual and visual sources, as well as from rich pre-existing common sense knowledge bases (e.g. ConceptNet, YAGO) and observations of relations between objects and concepts as they appear in visual and linguistic sources (i.e. images, videos, documents). The resulting CSR will be a dense, low-dimensional space that efficiently and accurately encapsulates hundreds of millions of observations. To enable more robust reasoning and question answering over this space than the state of the art, we will develop novel inference techniques for both transitive and multi-hop reasoning over the space (e.g. bats have wings, wings enable flying, thus, bats can fly). We apply our system to shared tasks and via an interactive demonstration system which supports direct questioning (e.g. Can the Grand Canyon fly to New York?). Our collaborators are Columbia University, UCLA, and UMass-Amherst. This research is based upon work supported by DARPA's MCS program, Contract N660011924032.
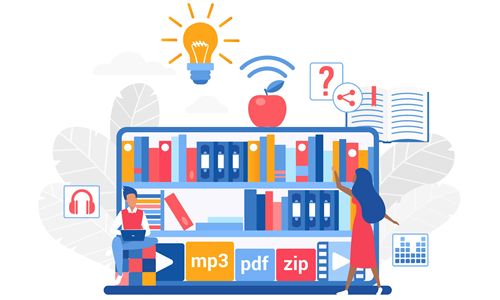
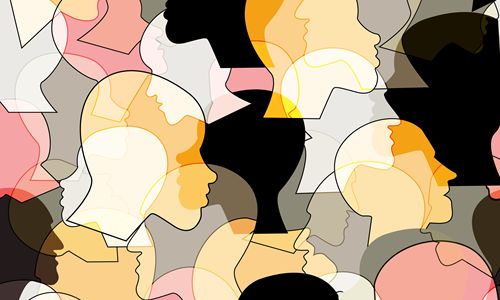
SARAL
Summarization and Domain-Adaptive Retrieval Across Languages
How can a monolingual English speaker access and understand text and speech material in low-resource foreign languages? In this project, we develop cross-lingual retrieval and summarization techniques that will work for any language in the world, given minimal resources to work with. Our collaborators are University of Notre Dame, Rensselaer Polytechnic Institute, Massachusetts Institute of Technology, Northeastern University, University of Massachusetts, and Idiap. This work is supported by the IARPA MATERIAL program. (Acknowledgement: This research is based upon work supported by the Office of the Director of National Intelligence (ODNI), Intelligence Advanced Research Projects Activity (IARPA), via AFRL Contract FA8650-17-C-9116.
Viola
Neural Generation with Improvised Dialogues and Common Sense Reasoning
The goal of the Alexa Prize Challenge is to build a social bot that can have a conversation with users that receives an average rating of 4.0+/5 and lasts more than 20 minutes. Our approach draws inspiration from improvisational theatre and common sense reasoning to build a social bot that is robust beyond the preset topics that bounded previous systems. This work is sponsored by Amazon.
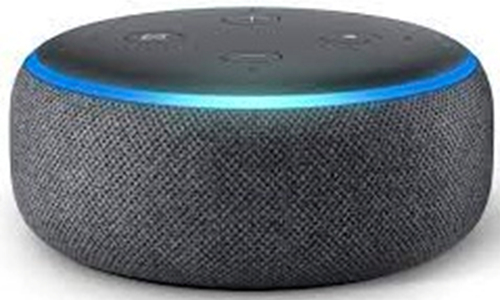