Latest News
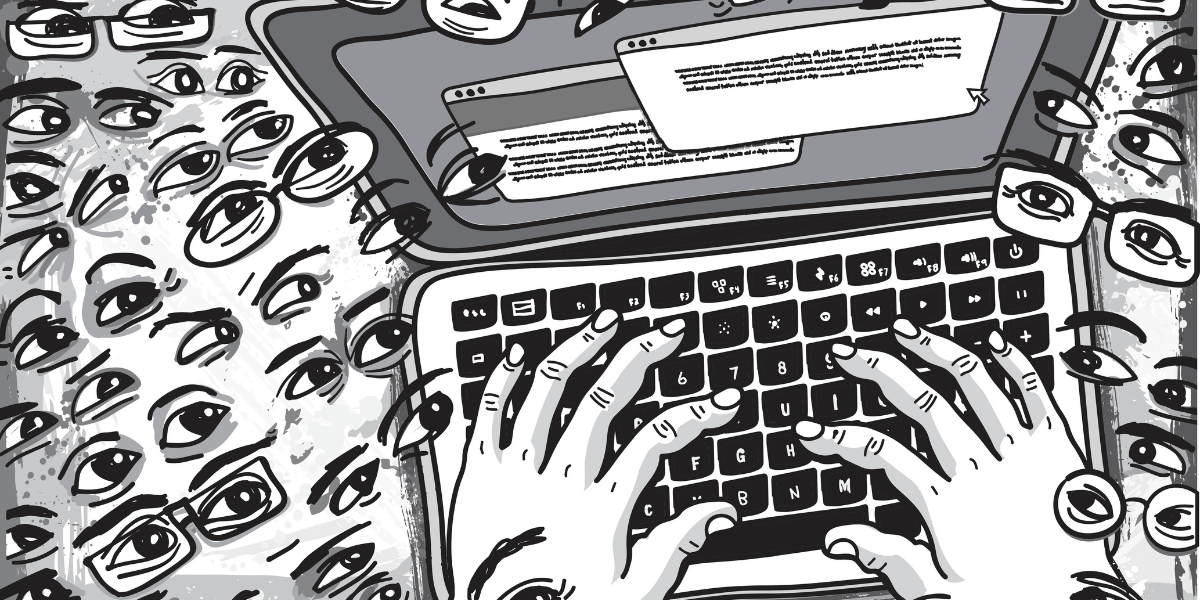
Uncovering Hidden Authors with AI
USC ISI computer scientists are developing language technologies that could potentially identify who wrote anonymous text.
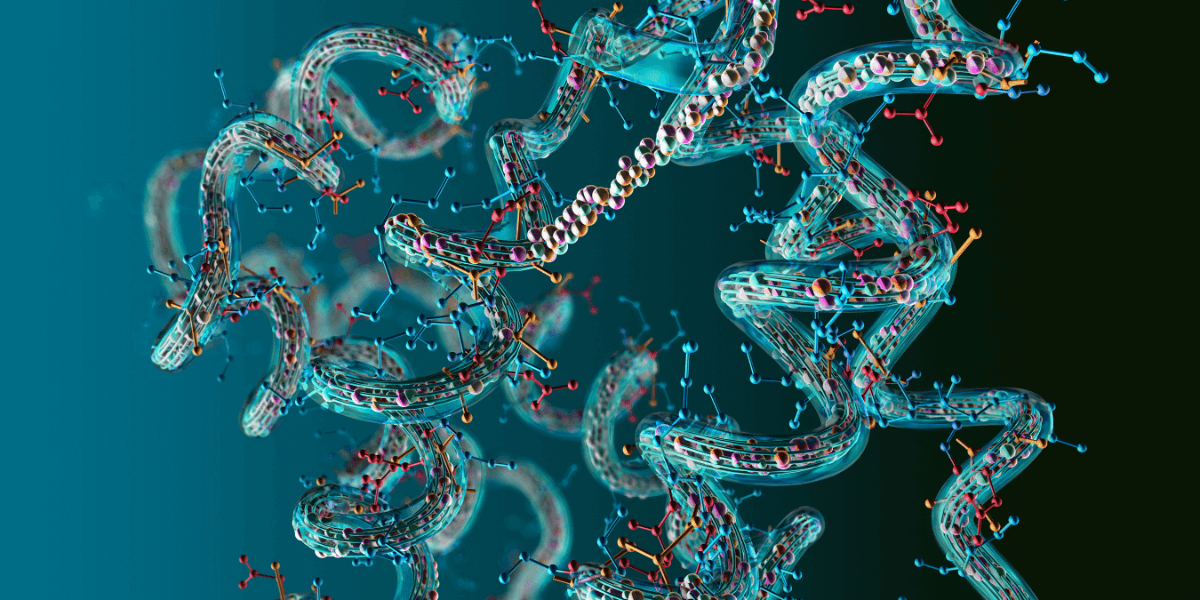
ISI Researchers Pioneer Developments In Protein Data Bank
ISI researchers are part of a core team developing the PDB-Dev repository, initiated by the Protein Data Bank, a foundational repository for macromolecular structures
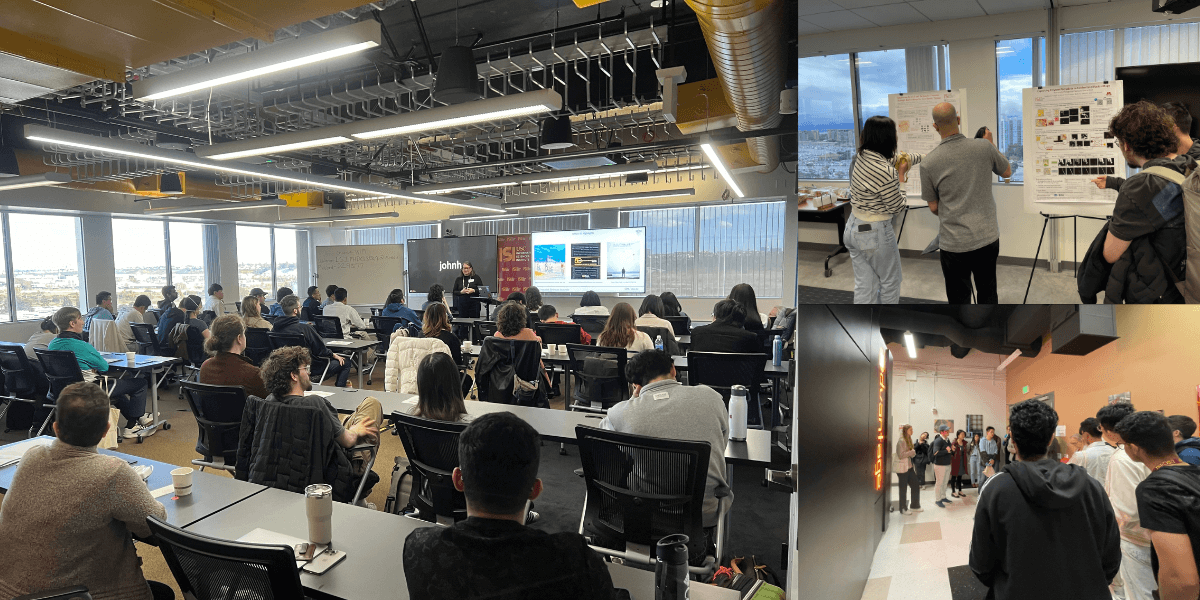
Inside ISI’s Ph.D. Visit Day 2024
Prospective graduate students traveled to ISI’s Marina Del Rey headquarters to experience the institute’s academic environment.
UPCOMING EVENTS
May
3
AI Seminar: Understanding LLMs through their Generative Behavior, Successes and Shortcomings
Swabha Swayamdipta, USC
-
May
9
NL Seminar-Event Extraction for Epidemic Prediction
Tanmay Parekh, UCLA
-
May
10
AI Seminar-Causal Inference to Inform Curation Practices in Online Platforms
Guiseppe Russo, EPFL- Ecole Polytechnique Fédérale de Lausanne
-