Latest News
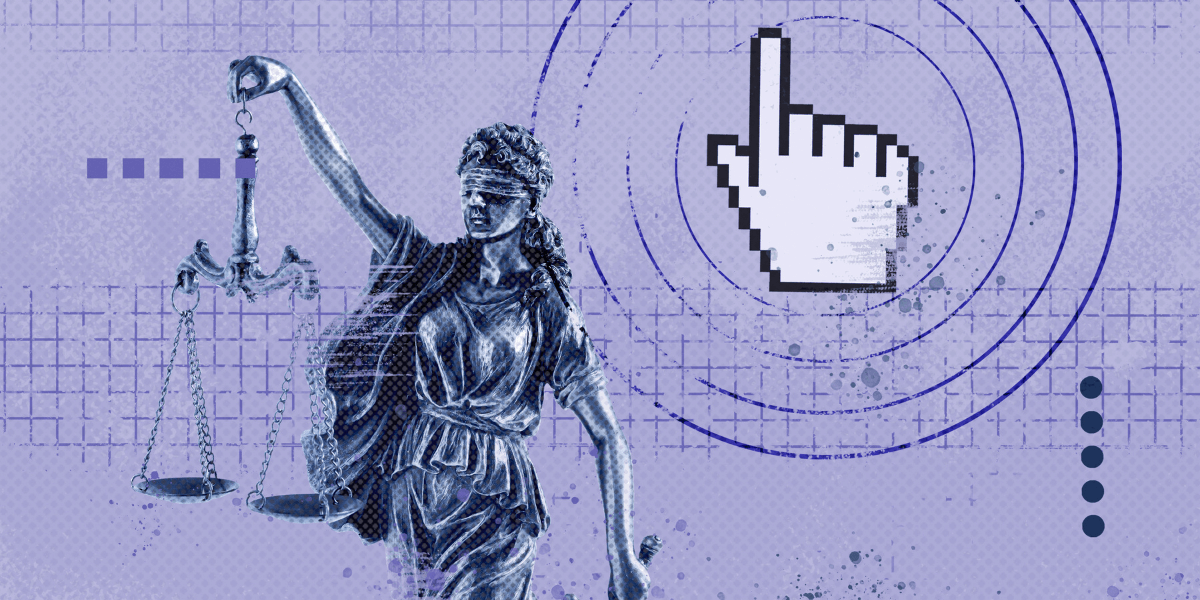
Making AI safe and fair
How ISI researchers are engaging in research, policy and thought leadership to guide AI toward positive futures.
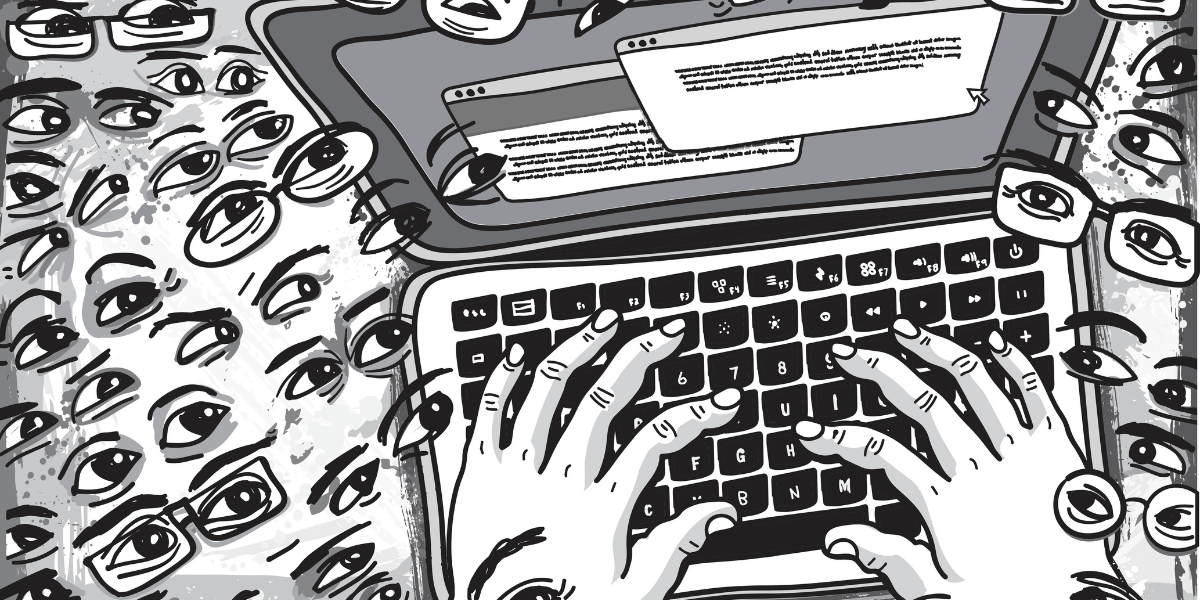
Uncovering Hidden Authors with AI
USC ISI computer scientists are developing language technologies that could potentially identify who wrote anonymous text.
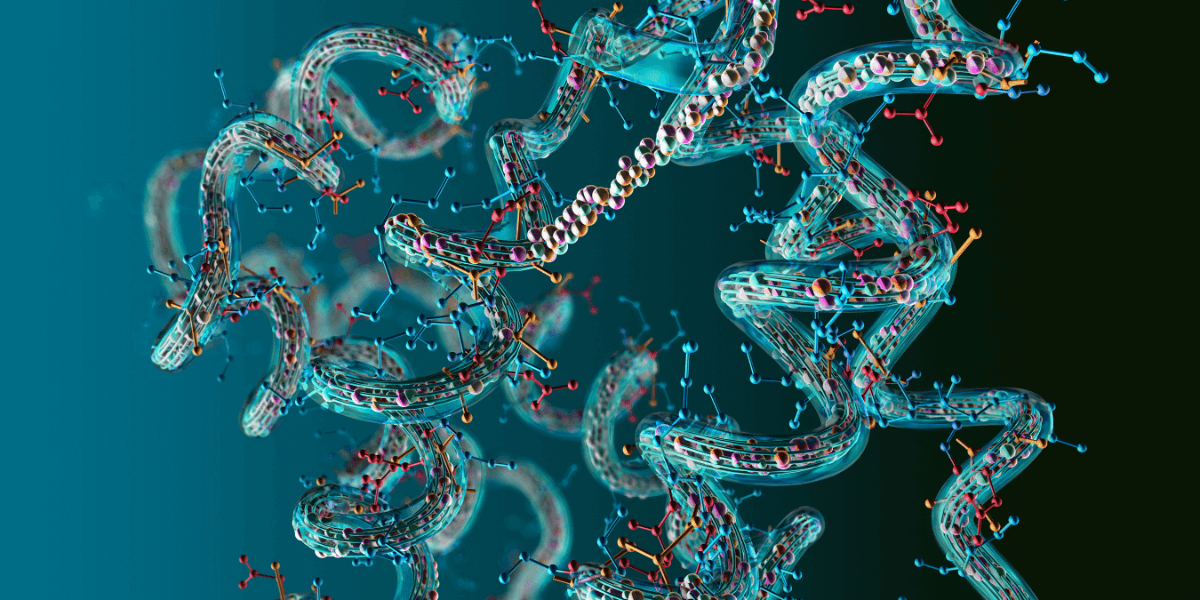
ISI Researchers Pioneer Developments In Protein Data Bank
ISI researchers are part of a core team developing the PDB-Dev repository, initiated by the Protein Data Bank, a foundational repository for macromolecular structures