Upcoming Seminars and Events
Unless otherwise noted, seminars are open to the public and free. You may also browse our past events.
Apr
26

May
3
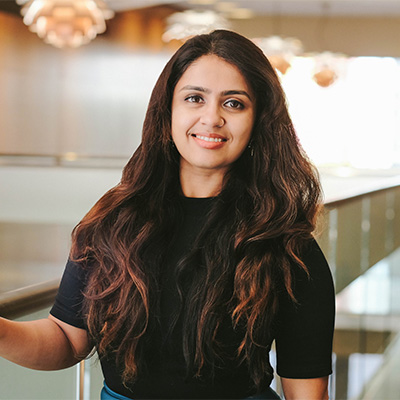
May
10
