Upcoming Seminars and Events
Unless otherwise noted, seminars are open to the public and free. You may also browse our past events.
May
14

May
17
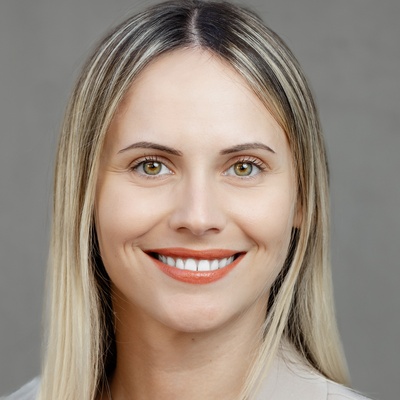
May
23
