Upcoming Seminars and Events
Unless otherwise noted, seminars are open to the public and free. You may also browse our past events.
May
3
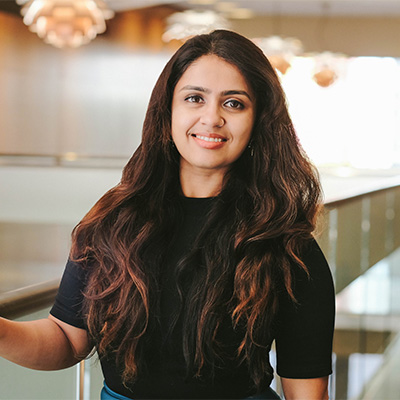
May
9
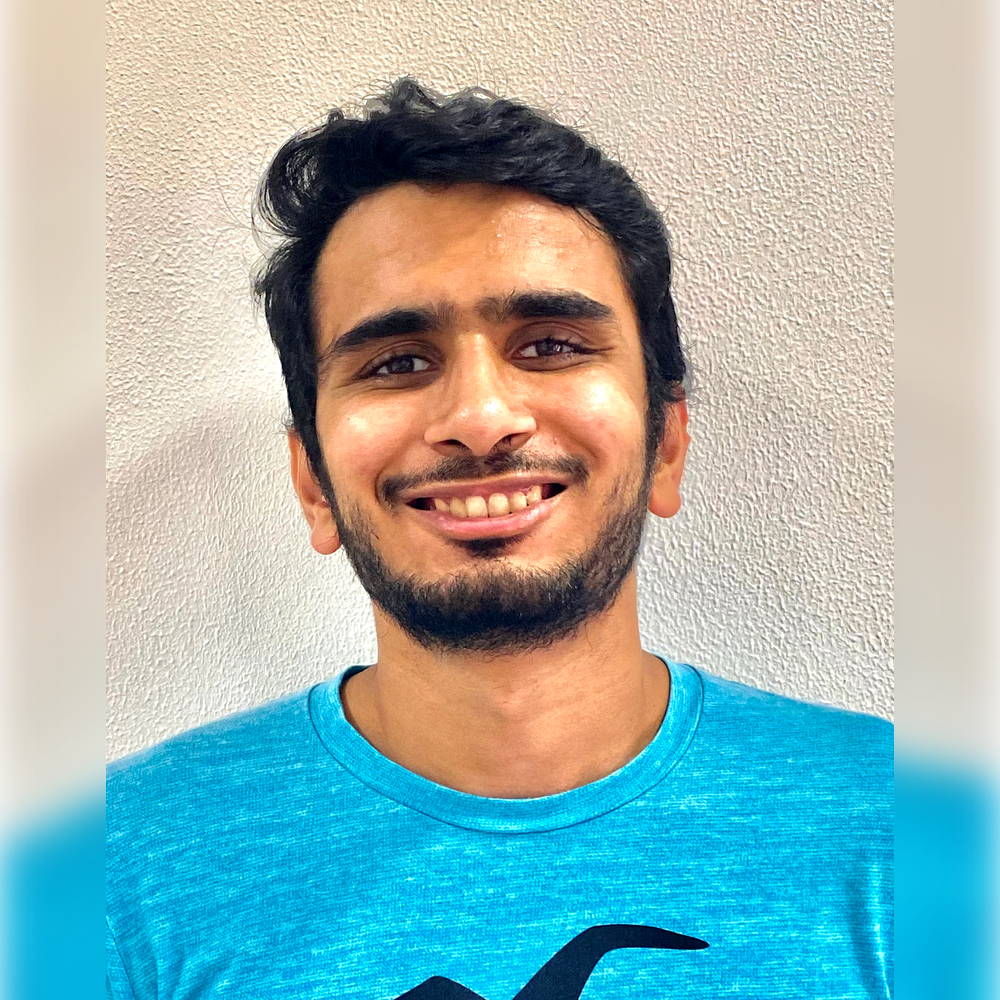
May
10

Unless otherwise noted, seminars are open to the public and free. You may also browse our past events.