ISI Directory
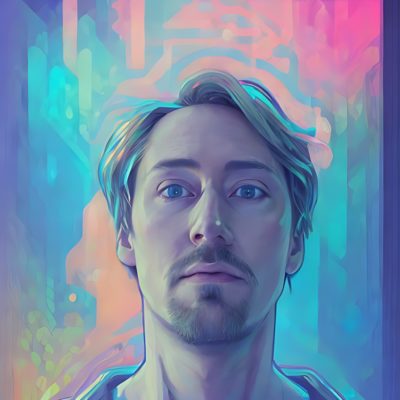
Greg Ver Steeg, Ph.D.
Education
Ph.D. Physics, Caltech
Bio
Starting 2023, I will be an associate professor at the University of California, Riverside. I will also maintain ties with USC colleagues and students as an adjunct research associate professor.
For more information and updates about me and my research, see my blog, google scholar page, or UCR website.