USC Researchers Present 12 Papers at AAAI
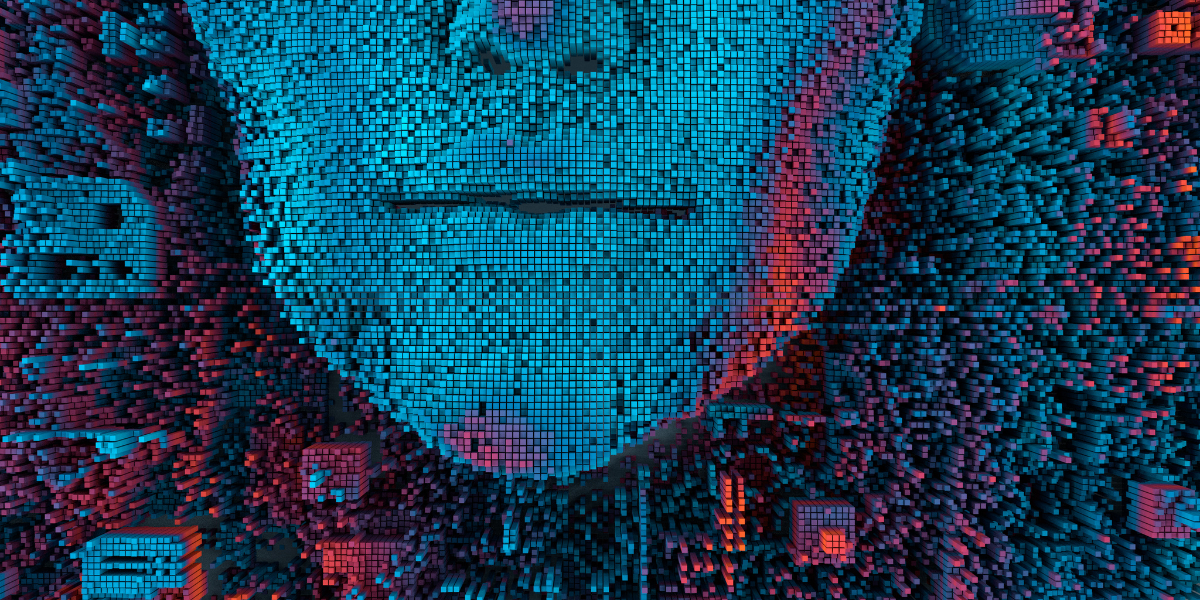
This year, USC researchers present 12 papers at the 37th AAAI Conference on Artificial Intelligence, solidifying the university’s position as a leader in AI research. Held in Washington, D.C., Feb. 7 to 14, the AAAI conference is one of the most prestigious forums for artificial intelligence research, attracting experts from around the world to discuss their latest findings.
Sven Koenig, a professor of computer science, received the AAAI Classic Paper Award 2023 for co-authoring a paper titled “Theta*: Any-Angle Path Planning on Grids.” The award recognizes papers that have made significant contributions to the field of artificial intelligence and have stood the test of time. The paper was first presented at the AAAI conference in 2007, and its lasting value has now been recognized over a decade later.
“Any-angle path finding” refers to a problem in robotics and computer science that involves finding the shortest path between two points on a grid-based map while allowing the path to deviate from the grid’s horizontal and vertical axes. The Theta* algorithm is capable of finding short and realistic-looking paths by propagating information only along grid edges and without constraining the paths, to achieve small runtimes and short paths.
The paper’s co-authors include Alex Nash and Kenny Daniel, who were Koenig’s students at the time of publication in the first semester of their PhD, and Ariel Felner, a visiting professor who is now department chair of the Department of Software and Information Systems Engineering at Ben-Gurion University. The research presented in the paper has had significant applications in various fields, including robotics, video games, and transportation planning.
The recognition of the paper as a test of time award emphasizes the long-term impact of Koenig and his team’s research and the continued relevance of their contributions to the field of artificial intelligence.
USC Papers at AAAI ’23
MAGIC: Mask-Guided Image Synthesis by Inverting a Quasi-Robust Classifier
Mozhdeh Rouhsedaghat (University of Southern California)*; Masoud Monajatipoor (UCLA); C.-C. Jay Kuo (USC); Iacopo Masi (Sapienza, University of Rome)
Layer-wise Adaptive Model Aggregation for Scalable Federated Learning
Sunwoo Lee (Inha University)*; Tuo Zhang (University of Southern California); Salman Avestimehr (University of Southern California)
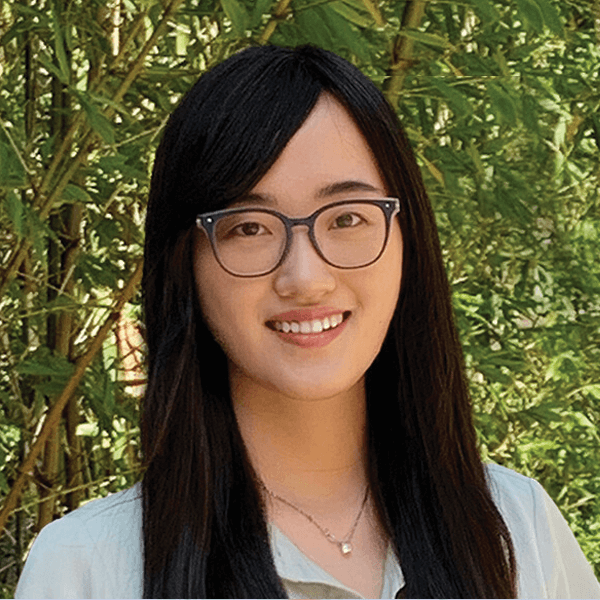
Research Spotlight: A future without traffic lights?
Intersection Coordination with Priority-Based Search for Autonomous Vehicles. Jiaoyang Li (pictured); The Anh Hoang; Zijun Lin; Hai L. Vu; Sven Koenig
The development of connected and autonomous cars provides an opportunity to manage road intersections without traffic signals, essentially by streams of cars passing through each other. While the future of signal-free intersections for autonomous cars holds promise for reducing traffic congestion and improving road safety, existing methods to manage such intersections are either too time-consuming to run in real-time or find solutions of bad quality. We develop a better method by building on recent multi-agent pathfinding technology to find near-optimal solutions.
Meta-Learning for Simple Regret Minimization
MohammadJavad Azizi (University of Southern California); Branislav Kveton (Amazon); Mohammad Ghavamzadeh (Google Research); Sumeet Katariya (UW-Madison and Amazon)
Overcoming Concept Shift in Domain-Aware Settings through Consolidated Internal Distributions
Mohammad Rostami (University of Southern California); Aram Galstyan (USC Information Sciences Institute)
The Role of Heuristics and Biases During Complex Choices with an AI Teammate
Nikolos Gurney (Institute for Creative Technologies, University of Southern California); John H Miller (CMU); David V Pynadath (USC)
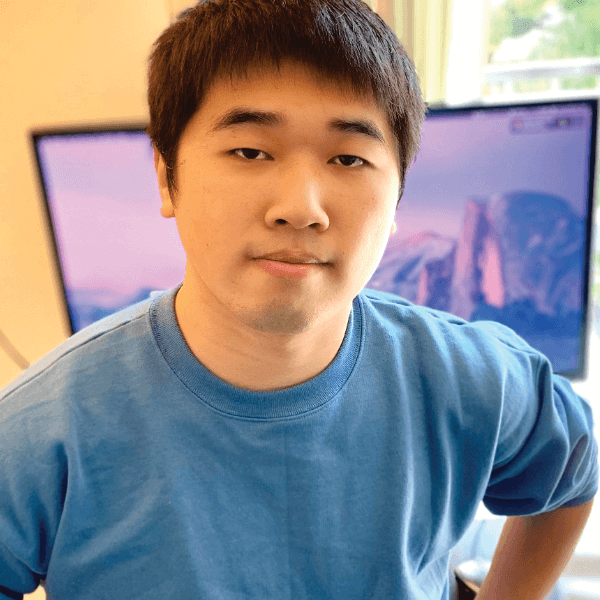
Research spotlight: Smarter robots for the home
On Grounded Planning for Embodied Tasks with Language Models. Bill Yuchen Lin (pictured); Chengsong Huang; Qian Liu; Wenda Gu; Sam Sommerer; Xiang Ren
Can robots be trained to perform household tasks in our homes? The ability to execute tasks in the real world is known as “embodied intelligence” in AI. Previous research has focused on building robotic agents that follow step-by-step instructions from humans. While these robots can perform well in simulated environments, they are limited by their dependence on human instructions. Is it possible to make robots smarter, so they can plan tasks independently? In this paper, we investigate using language models to plan tasks in real-world environments, with the goal of making robots more useful to humans in the future.
FairFed: Enabling Group Fairness in Federated Learning
Yahya H. Ezzeldin (University of Southern California); Shen Yan (University of Southern California); Chaoyang He (University of Southern California); Emilio Ferrara (University of Southern California, USA); Salman Avestimehr (University of Southern California)
Fairness in Contextual Resource Allocation Systems: Metrics and Incompatibility Results
Nathanael Jo (University of Southern California); Bill Tang (University of Southern California); Sina Aghaei (University of Southern California); Phebe Vayanos (University of Southern California); Eric Rice (University of Southern California); Kathryn Dullerud (University of Southern California)
Securing Secure Aggregation: Mitigating Multi-Round Privacy Leakage in Federated Learning
Ramy E. Ali (University of Southern California); Jinhyun So (University of Southern California); Basak Guler (University of California, Riverside); Jiantao Jiao (University of California, Berkeley); Salman Avestimehr (University of Southern California)
Solving Math Word Problems Concerning Systems of Equations with GPT3
Mingyu Zong, Bhaskar Krishnamachari
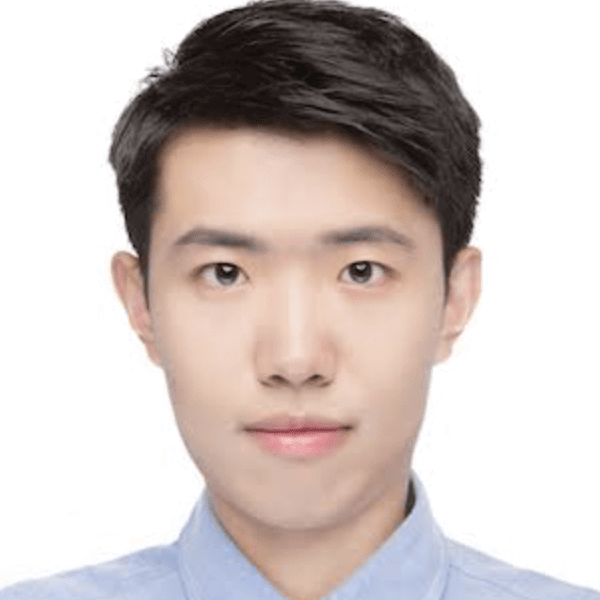
Research Spotlight: Unlocking the power of ‘What-If’ questions in healthcare
Estimating Treatment Effects from Irregular Time Series Observations with Hidden Confounders. Defu Cao (pictured); James Enouen; Yujing Wang; Xiangchen Song; Chuizheng Meng; Hao Niu; Yan Liu
We want to answer “what-if” questions to explore potential outcomes. For instance, in a healthcare setting, this could help medical professionals evaluate the potential consequences of clinical decisions, treatments and interventions. For example, what if a patient takes medication instead of surgery? Or, what if the patient doesn’t respond to the initial treatment? Our experiments demonstrate the potential of our model for healthcare applications in personalized medical recommendations to help doctors decide which treatment is best for a specific individual.
Published on February 6th, 2023
Last updated on May 16th, 2024